CHARTISTS AND TECHNICAL
ANALYSTS
I. Framework
II. The Basis of Technical Analysis
III. The Nature of Technical
Analysis
THE EMPIRICAL EVIDENCE ON SIMPLE PRICE
PATTERNS
- Investors have used price charts and price patterns as tools for predicting future price movements for as
long as there have been financial markets.
- The first studies of market efficiency focused
on the relationship between price
changes over time, to see if in fact
such predictions were feasible.
- Evidence can be classified into two
classes
- studies that focus on short-term (intraday,
daily and weekly price movements) price behavior and
- research that examines long-term (annual and
five-year returns) price movements.
a. Serial correlation
- Serial correlation measures the correlation between price changes in
consecutive time periods
- Measure of how much price change in any period
depends upon price change over prior time period.
- 0: imply that
price changes in consecutive time periods are uncorrelated with
each other
- >0:
evidence of price momentum in markets
- <0:
Evidence of price reversals
- From viewpoint of investment strategy,
serial correlations can be
exploited to earn excess returns.
- A positive serial
correlation would be exploited by a
strategy of buying after periods with positive returns and
selling after periods with negative returns.
- A negative serial
correlation would suggest a strategy
of buying after periods with negative returns and selling after
periods with positive returns.
- The correlations must be large enough for investors to
generate profits to cover transactions
costs.
Serial Correlation in Short-period
Returns
Author
|
Data
|
Variables
|
Time Interval
|
Correlation
|
Kendall & Alexander(28
|
19 indices - UK
|
price
|
1 week
2 weeks
4 weeks
|
0.131
0.134
0.006
|
Moore (28)
|
30 companies - US
|
log prices
|
1 week
|
-0.056
|
Cootner (28)
|
45 companies
US
|
log prices
|
1 week
|
-0.047
|
Fama (46)
|
30 companies - US
|
log prices
|
1 day
4 days
9 days
|
0.026
-0.039
-0.053
|
King (28)
|
63 companies - US
|
log prices
|
1 month
|
0.018
|
Niarchos (119)
|
15 companies - Greece
|
log prices
|
1 month
|
0.036
|
Praetz (128)
|
16 indices
20 companies
|
log prices
|
1 week
1 week
|
0.000
-0.118
|
Summary of Findings
- Serial correlations in most markets is
small. While there may be statistical
significance associated with these correlations, it is unlikely
that there is enough correlation to generate excess
returns.
- The serial correlation in short period returns
is also affected by price measurement
issues and the market micro-structure
characteristics.
- Non-trading
in some of the components of the index can create a
carry-over effect from the prior time period, this can result in
positive serial
correlation in the index
returns.
- The bid-ask
spread creates a bias in the
opposite direction, if transactions prices are used to compute
returns, since prices have a equal chance of ending up at the
bid or the ask price. The bounce that this induces in prices
will result in negative serial
correlations in returns.
Bid-Ask Spread = Square root of (Serial Covariance
in returns)
where the serial covariance in returns measures
the covariance between return changes in consecutive time periods.
b. Filter Rules
- In a filter rule, an
investor buys an investment if the price rises X% from a previous
low and holds the investment until the price drops X% from a
previous high. The magnitude of the
change (X%) that triggers the trades can vary from filter rule to
filter rule. with smaller changes resulting in more transactions
per period and higher transactions costs.
Figure 9.1: Illustration of Filter
Rule
Assumptions underlying
strategy
- This strategy is based upon the assumption that price changes are
serially correlated and that there is
price momentum, i.e., stocks which have gone up strongly in the
past are more likely to keep going up than go down.
- The following table summarizes results from a
study on returns, before and after transactions costs, on a
trading strategy based upon filter rules ranging from 0.5% to 20%.
( A 0.5% rule implies that a stock is bought when it rises 0.5%
from a previous low and sold when it falls 0.5% from a prior
high.)
Table 9.2: Returns on Filter Rule
Strategies
Value of X
|
Return with
strategy
|
Return with Buy and
Hold
|
# Transactions with
strategy
|
Return after transactions
costs
|
0.5%
|
11.5%
|
10.4%
|
12,514
|
-103.6%
|
1.0%
|
5.5%
|
10.3%
|
8,660
|
-74.9%
|
2.0%
|
0..2%
|
10.3%
|
4,764
|
-45.2%
|
3.0%
|
-1.7%
|
10.1%
|
2,994
|
-30.5%
|
4.0%
|
0.1%
|
10.1%
|
2,013
|
-19.5%
|
5.0%
|
-1.9%
|
10.0%
|
1,484
|
-16.6%
|
6.0%
|
1.3%
|
9.7%
|
1,071
|
-9.4%
|
8.0%
|
1.7%
|
9.6%
|
653
|
-5.0%
|
10.0%
|
3.0%
|
9.6%
|
435
|
-1.4%
|
12.0%
|
5.3%
|
9.4%
|
289
|
2.3%
|
10.3%
|
224
|
1.4%
|
16.0%
|
4.2%
|
Results of Study
- The only filter rule
that beats the returns from the buy and hold strategy is the 0.5%
rule, but it does so before
transactions costs. This strategy creates 12,514 trades during
the period which generate enough transactions costs to wipe out
the principal invested by the investor.
- While this test is an dated, it also
illustrates a basic problem with
strategies that require frequent short term
trading. Even though these strategies
may earn excess returns prior to transactions costs, adjusting for
these costs can wipe out the excess returns.
Relative Strength Rules
- A variant on the filter rule is the
relative strength
measure, which relates recent prices on
stocks or other investments to either average prices over a
specified period, say over six months, or to the price at the
beginning of the period.
- Stocks which score high on the relative strength
measure are considered good
investments.
- This investment strategy is also based upon
the assumption of price
momentum.
c. Runs Tests
- A runs test is a non-parametric variation on the serial
correlation, and it is based upon a
count of the number of runs, i.e., sequences of price increases or
decreases, in the price changes. Thus, the following price
changes, where U is an increase and D a decrease would result in
the following runs:
UUU DD U DDD UU DD U D UU DD U DD UUU DD UU D UU D
There were 18 runs in this price series of 33
periods.
- The actual number of
runs in the price series is compared against the number that can
be expected in a series of this length,
assuming that price changes are random.
- If the actual number of runs is
greater
than the expected number, there is evidence of negative correlation in
price changes.
- If it is
lower, there is evidence of
positive correlation.
Studies of Price Runs
- A study of price changes in the Dow 30
stocks, assuming daily, four-day, nine-day and sixteen day return
intervals provided the following results -
DIFFERENCING INTERVAL
Daily Four-day Nine-day Sixteen-day
Actual runs 735.1 175.7 74.6 41.6
Expected runs 759.8 175.8 75.3 41.7
- Based upon these results, there is
evidence of positive correlation in
daily returns but no evidence of
deviations from normality for longer return intervals.
- Long strings of positive and negative
changes are, by themselves,
insufficient evidence that markets are not random, since such behavior is
consistent with price changes following a random walk. It is the
recurrence of these strings that can be viewed as evidence against
randomness in price behavior.
SEASONAL AND TEMPORAL PATTERNS IN
PRICES
a. The January Effect
- Studies of returns in the United States and
other major financial markets consistently reveal strong
differences in return behavior across the months of the year.
Figure 9.13 reports average returns by month of the year from 1926
to 1983.
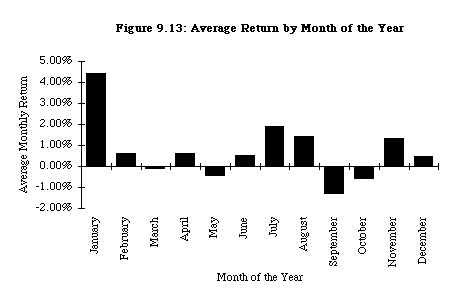
- Returns in January are significantly higher
than returns in any other month of the year. This phenomenon is called the year-end or January
effect, and it can be traced to the first two weeks in January.
- The January effect is much more accentuated for small firms than for larger firms, and roughly half of the small
firm premium, described in the prior section, is earned in the
first two days of January. Figure 9.14 graphs returns in January
by size and risk class for data from 1935 to 1986.
Figure 9.14: Returns in January by Size
and Risk Class - 1935-86
Explanations for the January
Effect
- A number of explanations have been advanced
for the January effect, but few hold up to serious scrutiny.
- Tax loss selling by
investors at the end of the year on
stocks which have 'lost money' to capture the capital gain,
driving prices down, presumably below true value, in December,
and a buying back of the same stocks in January, resulting in
the high returns.
- A second rationale is that the January
effect is related to institutional
trading behavior around the turn of
the years. It has been noted, for instance, that ratio of buys
to sells for institutions drops significantly below average in
the days before the turn of the year and picks to above average
in the months that follow.
Figure 9.15: Institutional
Buying/Selling around Year-end
Figure 9.16: Returns in January vs
Other Months - Major Financial Markets
b. The Weekend Effect
The weekend effect is another phenomenon that has
persisted over long periods and over a number of international markets. It refers to
the differences in returns between Mondays and other days of the
week. Figure 9.17, which graphs returns by days of the week from 1962
to 1978
.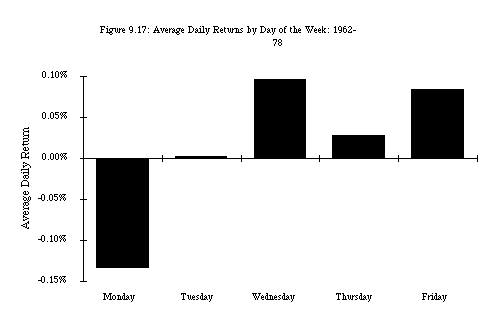
The Weekend Effect:
Explanations
- There are a number of other findings on the
Monday effect that have fleshed it out.
- First, the Monday effect is really a
weekend effect since the bulk of the negative returns is manifested
in the Friday close to Monday open
returns. The returns from intraday
returns on Monday are not the culprits in creating the negative
returns.
- Second, the Monday effect is
worse for small
stocks than for larger stocks.
- Third, the Monday effect is no worse following three-day weekends than two-day weekends.
- There are some who have argued that the
weekend effect is the result of bad news
being revealed after the close of
trading on Friday and during the weekend. Even if this were a
widespread phenomenon, the return behavior would be
inconsistent with a rational
market, since rational investors would
build in the expectation of the bad news over the weekend into the
price before the weekend, leading to an elimination of the weekend
effect.
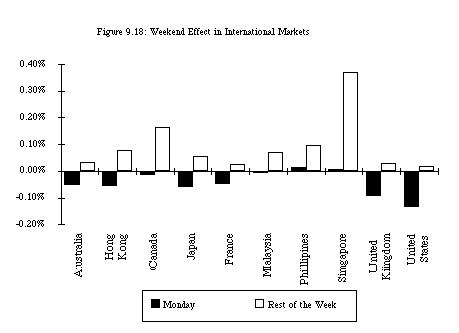
Further Notes on the Weekend
Effect
- The presence of a strong weekend effect in Japan, which allowed Saturday trading for a portion of the
period studies here indicates that there might be a more direct
reason for negative returns on Mondays than bad information over
the weekend.
- As a final note, the negative returns on
Mondays cannot be just
attributed to the absence of trading over the
weekend. The returns on days following trading holidays, in
general, are characterized by positive, not negative, returns.
Figure 9.19 summarizes returns on trading days following major
holidays and confirms this pattern.
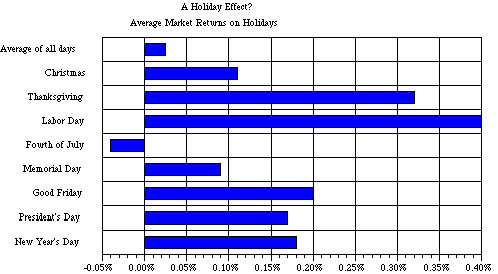
FOUNDATIONS OF TECHNICAL
ANALYSIS
A summary of the underlying assumptions
(Levy)
(1) Market value is
determined solely by the interaction of supply and demand
(2) Supply and demand
are governed by numerous factors both rational and
irrational. The market continually and
automatically weighs all these factors. (A random walker would have
no qualms about this assumption either. He would however point out
that any irrational factors are just as likely to be one side of the
market as on the other.)
(3) Disregarding minor
fluctuations in the market, stock prices tend to move in trends which
persist for an appreciable length of time. ( Random walker would disagree with this statement. For any
trend to persist there has to be some collective
'irrationality')
(4) Changes in trend are
caused by shifts in demand and supply. These shifts no matter why
they occur, can be detected sooner or later in the action of the
market itself. (In the financial
economist's view the market (through the price) will instantaneously
reflect any shifts in the demand and supply. However knowledge that
the demand or supply has shifted after it has already been reflected
in the price is worthless.)
On why technical analysts think it is
futile to estimate intrinsic values
"It is futile to assign an intrinsic value to a
stock certificate. One share of US Steel , for example, was worth
$261 in the early fall of 1929, but you could buy it for only $22 in
June 1932. By March 1937 it was selling for $126 and just one year
later for $38. ... This sort of thing, this wide deivergence between
presumed value and intrinsic value, is not the exception; it is the
rule; it is going on all the time. The fact
is that the real value of US Steel is determined at any give time
solely, definitely and inexorably by supply and demand, which are
accurately reflected in the transactions consummated on the floor of
the exchange.
Of course, the statistics which the
fundamentalists study play a part in the supply and demand equation-
that is freely admitted. But there are many other factors affecting
it. The market price reflects not only the differing fears and guesses and moods, rational and
irrational, of hundreds of potential buyers and sellers.. as well as
their needs and resources- in total,
factors which defy analysis and for which no statistics are obtainable but which
nevertheless are all synthesised, weighted and finally expressed in
the one precise figure at which a buyer and seller get together and
make a deal. This is the only figure that
counts.
THE RATIONALITY OF
INVESTORS
- Historians who have examined the behavior of
financial markets over time have challenged the assumption of rationality
that underlies much of efficient market theory.
- They point out to the frequency with
speculative bubbles have formed in
financial markers, as investors buy
into fads or get-rich-quick schemes, and the crashes with these
bubbles have ended, and suggest that there is nothing to prevent
the recurrence of this phenomenon in today's financial markets.
There is some evidence in the literature of irrationality on the
part of market players.
a. Experimental Studies of
Rationality
- While most experimental studies suggest
that traders are rational, there are some examples of irrational
behavior in some of these studies.
- One such study was done at the University of
Arizona. In an experimental study, traders were told that a
payout would
be declared after each trading day, determined randomly from four
possibilities - zero, eight, 28 or 60
cents. The average payout was 24 cents.
Thus the share's expected value on the first trading day of a
fifteen day experiment was $3.60 (24*15), the second day
was $3.36 .... The traders were allowed to trade each day. The
results of 60 such experiments is summarized in the following
graph.
Trading Price by Trading Day
Results of Experimental
Study
- There is clear evidence here of a
'speculative bubble' forming during periods 3 to 5, where prices exceed
expected values by a significant amount.
- The bubble
ultimately bursts, and prices approach
expected value by the end of the period.
- If this is feasible in a simple market, where
every investor obtains the same information, it is clearly feasible in real financial
markets, where there is much more
differential information and much greater uncertainty about
expected value.
- Some of the experiments were run with
students, and
some with Tucson
businessmen, with 'real world'
experience. The results were similar for both groups.
- Furthermore, when price curbs of 15 cents were
introduced, the booms lasted even
longer because traders knew that prices
would not fall by more than 15 cents in a period. Thus, the notion
that price limits can control speculative bubbles seems
misguided.
b. Chaos and Non-linear Dynamics in
Prices
- The recent use of chaos theory to explain
natural phenomena (such as weather patterns) has also generated
some interest into its potential uses in explaining stock price
behavior.
- Peters, for instance, has tried to
model price behavior using the non-linear
models that underlie much of chaos
theory with mixed results.
A GLOSSARY OF NEW AGE
TECHNOLOGY
- Artificial Intelligence: Software systems that attempt to replicate aspects of human intelligence.
- Expert System:
Computerized decision-making technique that embodies
knowledge gleaned from
experts.
- Neural Network:
Tries to mimic human brain
processes and learns from mistakes it
makes.
- Chaos Theory:
Holds that seemingly random event s
actually have patterns that computers
can detect.
Genetic Algorithm:
Problem solving technique useful in identifying and handling
anomalies. Evolution is used to find winners.
I. MARKET OVERREACTION: The Contrarian
Indicators
Basis: Research in
experimental psychology suggests that people tend to overreact to
unexpected and dramatic news events. In revising their beliefs,
individuals tend to overweight recent information and underweight
prior data.
Empirical evidence:
If markets overreact then
(1) Extreme movements in stock prices will be
followed by subsequent price movements in the opposite
direction.
(2) The more extreme the price adjustment, the
greater will be the subsequent adjustment
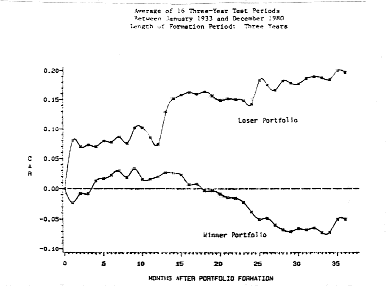
Issues: (1) Why, if
this is true, is is that contrarian investors are so few in number or
market power that the overreaction to new information is allowed to
continue for so long?
(2) In what sense does this phenomenon justify th
accusation that the market is inefficient?
(3) Is the market more efficient about
incorporating some types of information than others?
Technical trading rules: Contrarian
Opinion
1. Odd-lot trading:
The odd-lot rule gives us an indication of what the man on the street
thinks about the stock (As he gets more enthusiastic about a stock
this ratio will increase).
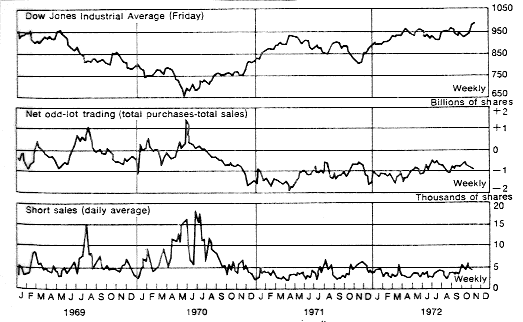
2. Mutual Fund Cash
positions: Historically, the argument
goes, mutual fund cash positions have been greatest at the bottom of
a bear market and lowest at the peak of a bull market. Hence
investing against this statistic may be profitable.
3. Investment Advisory opinion: This is the ratio of advisory services that are bearish.
When this ratio reaches the threshold (eg 60%) the contrarian starts
buying.
II. DETECTING SHIFTS IN DEMAND AND
SUPPLY USING PAST PRICE CHANGES: The Lessons in Price
Patterns
The Shiller Effect:
The true value is the present value of all expected future dividends.
However the variance in current prices is substantially greater than
the variance in this present value.
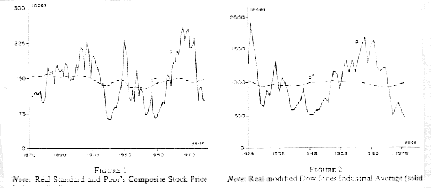
Technical rules: Breadth of the
market
Measure: This is a
measure of the number of stocks in the market which have advanced
relative to those that have declined. The broader the market, the
stronger the demand.
Related measures:
(1) Divergence between different market indices (Dow 30 vs NYSE
composite) (2) Advance/Decline lines
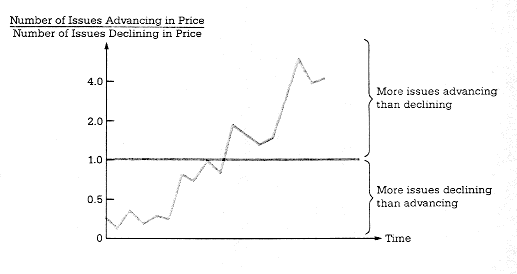
(2) Support and Resistance
lines: A common explanation given by
technicians for market movements is that markets have support and
resistance lines. If either is broken, the market is poised for a
major move.
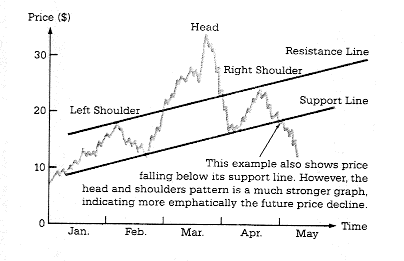
Possible rationale:
(1) Institutional buy/sell programs which can be triggered by
breakthrough of certain well defined price levels (eg. Dow 1300) (2)
Self fulfilling prophecies: How money managers use technical analysts
for window dressing.
(3) Moving
Averages: A moving average line smooths
out fluctuations and enables the chartist to see trends in the stock
price. How that trend is interpreted then depends upon the
chartist.
(4) Trading Volume
Indicators: Some technical analysts
believe that there is information about future price changes in
trading volume shifts.
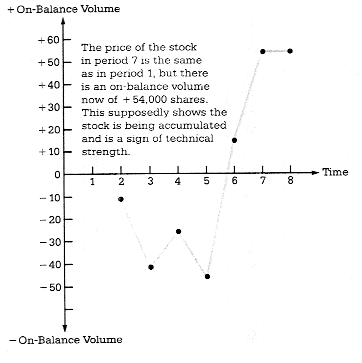
(5) Point and figure
charts:
III. MARKETS LEARN SLOWLY: The Momentum
Investors
Basis:
The argument here is that markets learn
slowly. Thus, investors who are a little quicker than the market in
assimilating and understanding information will earn excess returns.
In addition, if markets learn slowly, there will be price drifts
(i.e., prices will move up or down over extended periods) and
technical analysis can detect these drifts and take advantage of
them.
The Evidence: There
is evidence, albeit mild, that prices do drift after significant news
announcements. For instance, following up on price changes after
large earnings surprises provides the following evidence.
Note the price drift, especially after the most
extreme earnings announcements.
Relative Strength rules: The relative strength of a stock is the ratio of its
current price to its average over a longer period (eg. six months).
The rule suggests buying stocks which have the highest relative
strength (which will also be the stocks that have gone up the most in
that period).
IV. MARKETS ARE CONTROLLED BY EXTERNAL
FORCES: The Mystics
The Elliot Wave:
Elliot's theory is that the market moves in waves of various sizes,
from those encompassing only individual trades to those lasting
centuries, perhaps longer. "By classifying these waves and counting
the various classifications it is possible to determine the relative
positions of the market at all times". "There can be no bull of bear
markets of one, seven or nine waves, for example.
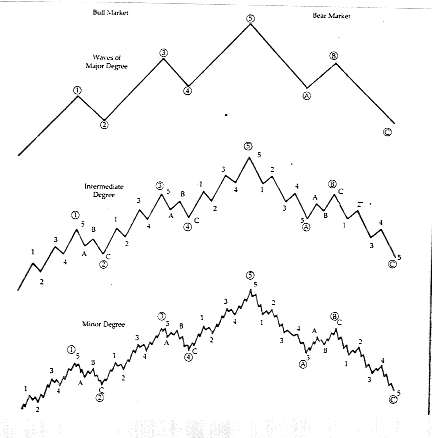
The Dow Theory:" The
market is always considered as having three movements, all going at
the same time. The first is the narrow movement (daily fluctuations)
from day to day. The second is the short swing (secondary movements)
running from two weeks to a month and the third is the main movement
(primary trends) covering at least four years in its duration.
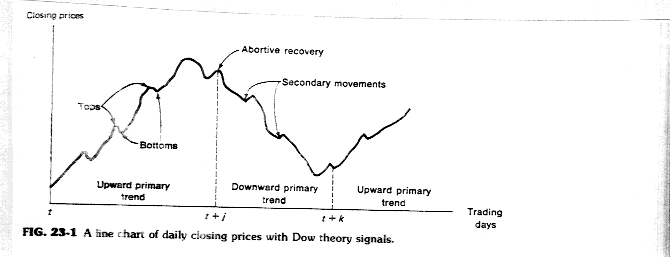
Price Patterns
V. FOLLOWING THE SMART INVESTORS: The
Followers
(a) Confidence
Index: The confidence index measures the
ratio of yields on risky bonds (eg. BBB) to safer bonds (AAA). A
higher ratio here is bullish.
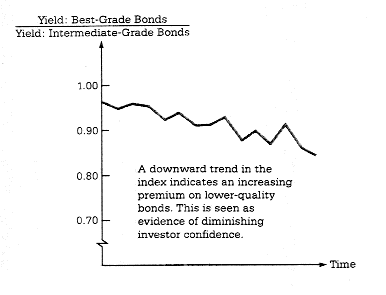
(b) Specialists' short
sales ratio: Specialists are better
informed than the average investor. an increase in their short sales
is considered a bearish sign.